https://www.brookings.edu/articles/generative-ai-the-american-worker-and-the-future-of-work/
Crimson Reason
A site devoted mostly to everything related to Information Technology under the sun - among other things.
Friday, October 11, 2024
Tuesday, October 8, 2024
Loss of Confidence in IT
This article very much jives with my own experiences and observations as a Senior Software Developer at GM IT during my years with General Motors. What this article fails to mention, which I experienced at GM IT, is the Fear and Hierarchy that permeated that entire IT organization.
At GM, the loss of confidence by the Business leaders in IT manifested itself in the creation and staffing of an entirely new GM IT facility in Mountainview and the reduction of GM IT in other GM IT locations by 2300 persons in about a year.
Failure always has consequences.
Thursday, October 3, 2024
Tuesday, October 1, 2024
Wednesday, September 25, 2024
AI and Philosophy
We face many problems and issues for which we would like to have answers or even partial answers.
We also available to us the works of all Thinkers and Philosophers of Europe and Western Asia written over the last 2500 years.
I think it will bea good idea to develop (train) Large Language Models for Plato, Aristotle, Ibn Sina, Ibn Rusd, Saint Thomas of Aqinas, and others. In this manner, we could pose questions to them, in a metaphoric way, and get their answers. Needless to say, such LLMs need to include our contemporary context as well, the Revolutionary changes that Empirical Sciences have caused in our understandings of the world as well as archaeological and historical knowledge that has been gained over the last 200 years.
Furthermore, such an approach may be extended to the very large corpus of religious commentaries and expositions of extant religions; in Judaism, Christianity, Islam, Hindu Spiritual Doctrines, and Buddhism.
Ideally, one could pose moral, metaphysical, epistemological, ontological questions to these LLMs and see what they come up with. They are meant as hypothesis generators and discussion starters and not a substitute for Thinkers and Philosophers.
News of IBM (and AI)
https://www.theregister.com/2024/09/24/ibm_layoffs_ai_talent/
We read ""Senior software engineers stopped being developed in the US around 2012... No country on Earth is producing new coders faster than old ones retire. India and Brazil were the last countries and both stopped growing new devs circa 2023. China stopped growing new devs in 2020."
Yet no employers have been begging me to join their organization!
I must be missing something crucial.
Monday, September 23, 2024
Tuesday, September 10, 2024
Pointing a User Story
When one attempts to point a User Story, one needs to take into account the 3 major factors pertaining to the delivery and completion of that User Story: Viz. Complexity, Effort, Uncertainty, in order to arrive at the points for it.
Factors for Estimating a User Story |
One way of doing so would be to point each of the 3 factors above separately, using the usual Fibonacci sequence to assign an independent value to each factor.
<script>
function GetSelectedValue() {
var complexity = $('#sel-options-complexity').val();
var effort = $('#sel-options-effort').val();
var uncertainty = $('#sel-options-uncertainty').val();
var score = Math.cbrt(complexity * effort * uncertainty);
score = fitToFibonacciNumber(score);
document.getElementById("combined-points").innerHTML = score;
}
function fitToFibonacciNumber(value) {
if (value >= 1 && value < 2) return 1;
if (value >= 2 && value < 3) return 2;
if (value >= 3 && value < 5) return 3;
if (value >= 5 && value < 8) return 5;
if (value >= 8 && value < 13) return 8;
if (value >= 13 && value < 21) return 13;
if (value >= 21 && value < 34) return 21;
if (value >= 34) return Math.trunc(value);
}
</script>
The method GetSelectedValue() would be invoked every time the user selects a value from any of the dropdowns. The result is then displayed on the UI (not shown here).
In principle, any other non-linear function could be used, such as logarithm, or even the Sigmoid function. One has to experiment with a stable Agile team over time to determine suitable alternatives to the geometric mean, utilized here.
In this treatment, the 3 factors Complexity, Effort, Uncertainty have been treated with equal weights. However, there could be situations in which the Agile team is tackling User Stories that are more uncertain (ambiguous) or are working with lower-than-needed staffing levels or are dealing with very complex User Stories. Under such circumstances, it might make sense to assign a different weight to each factor and then calibrate the weights over several Sprints with the Agile team.
Thursday, September 5, 2024
News of Cyborgs
In a case of Life imitating Art, we learn of a cyborg whose biological component is a fungus:
https://www.cnn.com/2024/09/04/science/fungus-robot-mushroom-biohybrid/index.html
Sunday, September 1, 2024
Programmer Joke
Question: How can you tell when you are talking to an extrovert programmer?
Answer: He stares at your shoes, when he talks to you!Thursday, August 29, 2024
Wednesday, August 28, 2024
Tuesday, August 27, 2024
Sunday, August 25, 2024
Analyzing Code with ChatGPT
I wonder if this approach could augment or replace such things as Profilers or (SQL) Execution Plans.
Saturday, August 24, 2024
Using AI for Software Maintenance
As someone who has used ChatGPT & Copilot for migrating a suite of Spark Streaming applications last year from Java 8 to Java 17, as well as for addressing GitHub Dependabot issues, I can attest to the plausibility of this report:
Friday, August 23, 2024
Data Monetization Estimation
William Thomson, Lord Kelvin |
Lecture on "Electrical Units of Measurement" (3 May 1883), published in Popular Lectures Vol. I, p. 73
Estimating Data Value
Introduction
There has been a lot of interest in data monetization over
the last decade or so and this discussion is meant as a way of thinking about
estimating the potential value of data and in contradistinction to realization
of that value. Think of this as an
analogue of a geological survey without any guarantee of finding economically
viable mines; an attempt at a quantitative analysis of what value data have
along the lines that the late Lord Kelvin had suggested.
Column Value Model
We assume a
company’s value to be a combination of human, physical, and data capital; a
fully automated company with no human resources is a pipedream, a company must
have physical assets to conduct business (be they rented) and must make
decisions based on some information or other.
(For simplicity, Goodwill valuation is excluded from this model).
So we start
with the following formula for the company’s valuation and the proceed to give
an estimate of its data assets in a more defined manner.
Company Valuation = Human Capital + Physical Capital +
Data Capital
Assume all
three parts have the same value and the company’s valuation is $ 3 billion:
Data Capital = Company Valuation/3 = $ 1 Billion
In this
model, we would like to estimate the average value of each column of data in
all the relational schemas that the company uses, i.e.
Let us
further assume that there are 50 relational schemas in this company, that they
each have 50 tables of 20 columns each.
This gives a total of 50,000 columns in total that yields an average
value of $ 20,000 per column. In this
approach, all columns are considered to be of equal value; be they customer
names (let us say) or such ubiquitous columns as “Last Updated Date”.
We can then
proceed to estimate an Average value for each Schema
Alternative Models
Data Volume Model
In this model, the value of each schema is estimated based
on its data volume. That is:
The total Data Valuation will be divided by this number, an average value per Gigabyte is extracted, and the value of each schema is then computed by multiplying its data volume by that average number.
Time Dependent Model
Another model is one with time-dependent variable weights
for value of each part of the company’s valuation, i.e.:
Valuation = h(t) Human Capital + p(t) Physical Capital + d(t)
Data Capital
With
constrains:
h(t) + p(t) + d(t) = 1 and h(t) ≥ 0, p(t) ≥ 0, d(t) ≥ 0
Weighted Schema Model
In this
model, we are capturing the importance of each schema – via the factor a(n) –
and the historical data volume available for each Schema by the second sum and
the factor b(m).
The exp(1-m)
is intended to model the aging and staleness of the data.
Useful Links
- .Net Code Samples
- AJAX for MS Developers
- C# Tutorials
- Channel9
- Code Search Engine
- Douglas Crockford's JavaScript Site
- DZONE
- Google Code
- IBM Developer Works
- IBM Public Skunkworks
- Is This Thing On?
- Java tutorials, hints, tips
- Jon Udell Weblog
- Knowing .Net
- Massive List of Information for Programmers
- MIT Courses
- MSDN
- Simple-Talk
- SUN Java
- That Indigo Girl
- UC Berkeley Lectures
- Yahoo UI Library
Topics
- 3-D Printing (13)
- AI (220)
- Art (93)
- Article (119)
- book (11)
- books (83)
- Business Intelligence (18)
- Careers (67)
- Cloud Computing (19)
- Cognition (13)
- Complexity (8)
- Computer Science (20)
- COVID-19 (1)
- Cyber-security (77)
- Data Analysis (39)
- Data Management (19)
- Data Visualization (30)
- Design Thinking (1)
- Embedded Tools (34)
- Gadgets (74)
- Games (32)
- Google (7)
- Hardware (39)
- High Performance Computing (32)
- History of Mathematics (1)
- Humor (73)
- Inetrview (7)
- Intelligent Transportation (17)
- IoT (15)
- IT as Metaphor (2)
- Magazine Subscription (8)
- Mathematics Tools (4)
- Microsoft Platforms (22)
- Microsoft Tools (63)
- Mobile Computing (2)
- Motto (3)
- Network Tools (12)
- News (118)
- Offshoring (6)
- Open-Source Sofware (7)
- Outsourcing (1)
- Philosophy (5)
- Pictures (141)
- PLM (5)
- Programming Languages (74)
- Quantum Computing (5)
- Reports (50)
- RFID (3)
- Robo (2)
- Robots (102)
- Science (57)
- Scientific Computing (17)
- Search Tools (7)
- Semantic Networks (11)
- Simulations (34)
- Social Computing (25)
- Software Architecture (27)
- Software Development (151)
- Software Testing (4)
- Software Tools (268)
- Some Thoughts (44)
- Speech (6)
- Standards - Telematics (9)
- Transportation (14)
- Video (11)
- Visualization (9)
- Web Site (223)
- Web Site for Science (48)
About Me
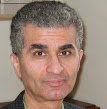
- Babak Makkinejad
- I had been a senior software developer working for HP and GM. I am interested in intelligent and scientific computing. I am passionate about computers as enablers for human imagination. The contents of this site are not in any way, shape, or form endorsed, approved, or otherwise authorized by HP, its subsidiaries, or its officers and shareholders.
Blog Archive
- October (4)
- September (7)
- August (11)
- July (6)
- June (11)
- May (12)
- April (7)
- March (5)
- February (1)
- January (3)
- December (1)
- October (2)
- September (4)
- August (1)
- July (3)
- June (2)
- April (2)
- March (2)
- February (2)
- January (10)
- December (1)
- October (1)
- September (1)
- August (4)
- June (1)
- April (6)
- March (2)
- February (4)
- January (3)
- December (1)
- October (1)
- June (3)
- April (1)
- March (1)
- February (1)
- January (6)
- December (8)
- November (3)
- October (5)
- September (2)
- August (3)
- July (6)
- June (2)
- May (7)
- April (19)
- March (22)
- February (6)
- January (5)
- December (4)
- November (4)
- October (9)
- September (3)
- August (7)
- July (3)
- June (2)
- May (6)
- April (4)
- March (8)
- February (5)
- January (18)
- December (6)
- November (10)
- October (6)
- September (7)
- August (2)
- July (4)
- June (5)
- May (8)
- April (5)
- March (9)
- February (3)
- January (7)
- December (2)
- November (1)
- October (3)
- September (5)
- August (10)
- July (8)
- May (5)
- April (8)
- March (9)
- February (6)
- January (11)
- November (6)
- October (9)
- September (5)
- August (13)
- July (9)
- June (9)
- May (8)
- April (4)
- March (2)
- February (8)
- January (9)
- December (3)
- November (7)
- October (9)
- September (7)
- August (4)
- July (2)
- June (4)
- May (7)
- March (4)
- February (2)
- January (1)
- December (2)
- November (1)
- October (6)
- September (1)
- August (1)
- July (4)
- June (1)
- April (1)
- March (1)
- February (1)
- January (2)
- December (5)
- October (4)
- August (2)
- July (3)
- June (8)
- May (7)
- April (5)
- March (9)
- February (3)
- January (7)
- December (4)
- October (7)
- September (5)
- August (5)
- July (8)
- June (6)
- May (9)
- April (5)
- March (4)
- February (5)
- January (6)
- December (12)
- November (7)
- October (5)
- September (4)
- August (19)
- July (12)
- June (4)
- May (8)
- April (5)
- March (15)
- February (5)
- January (9)
- December (14)
- November (6)
- October (12)
- September (2)
- August (10)
- July (8)
- June (8)
- May (11)
- April (10)
- March (10)
- February (9)
- January (20)
- December (16)
- November (9)
- October (25)
- September (24)
- August (12)
- July (18)
- June (20)
- May (13)
- April (29)
- March (26)
- February (14)
- January (17)
- December (17)
- November (9)
- October (32)
- September (27)
- August (27)
- July (11)
- June (22)
- May (25)
- April (33)
- March (33)
- February (28)
- January (38)
- December (12)
- November (39)
- October (28)
- September (29)
- August (29)
- July (18)
- June (27)
- May (17)
- April (23)
- March (40)
- February (31)
- January (6)