A site devoted mostly to everything related to Information Technology under the sun - among other things.
Sunday, December 29, 2024
Saturday, December 28, 2024
Geoffrey Hinton on AI Future
Dr. Geoffrey Hinton is articulating a pretty scary scenario of the AI future, could he be right?
I do not think so, he is wrong on many levels.
(I wonder why these physicists always are full of Gloom & Doom; like Steven Hawking predicting the demise of man because of Global Warming.)
AI is not smarter than humans, it contains a slice of human rationality which is codified into an electronic machine. He would be righter if he could point out to machines that are capable of Insight. Insight is a very complex human capability and, IMHO, like Consciousness, is a Mystery.
I worked with AI/ML scientists here at GM and the neural nets that they were using required training data sets that, over millions of data sets (e.g. pictures of dogs and cats), would cause them to recognize patterns. I understood the basic concepts and structures; there was no deep mystery there. But, our AI, for recognizing a Traffic Stop Sign, could be fooled by sticking a rectangular reflecting strip on the sign.
I have no idea how ChatGPT or Gemini, which are examples of Large Language Models, are constructed. I think they are based on what has been accomplished in Natural Language Processing. NLP started with mathematical statistics, but I am ignorant as to what additional techniques are used in it since the machine translations are adequate now but are not great. The key ingredient, however, is still the prior human corpus for the machines to use. In case of LLMs, they lie, and they also respond to corrective algorithms by trying to neutralize them. (Please see here: Crimson Reason: Frontier Models are Capable of In-context Scheming.) I asked ChatGPT for a book on Assyria and it gave me titles that did not exist!
An LLM for Swahili, or any of the Bantu languages, for which no large corpus exists will be useless. The reasons that LLM-based system is so impressive is the existence of large corpuses that have the codified human knowledge in multiple languages.
Isn't this rather worrying if the intention is for AI to replace human workers? I mean, if a system integrates lying and scheming into its strategy and the kind of data it gives to its handlers, this is worrying. In truth, the AI system doesn't know what the truth is. Should it know that it should value the truth, though, but is that possible for it to know if it doesn't fully understand what 'truth' is? And of course, one could argue that we, as humans, do not agree as to what the 'truth' is. But searching for Truth, in all aspects of human endeavor, is very much part of our mental and emotional life; "What is the Truth?". That question could not be formalized, IMHO; we already have proof of that in Godel's Incompleteness Theorem.
Google has an AI tool integrated into its search
engine, and you can see how it works. It's basically a crawler that collates
information from across the web. On occasions, I've noticed it comes up with
totally unreliable data. I am going to country X for a holiday, and I
was checking on visas, etc. It came up with old and/or misleading data, because
the rules have changed and it was probably using data posted up on amateurish
blogs etc. posted up by travellers who may not be that well informed, and so on.
Replicated on a larger scale and over more important issues, such as medicine or finance, this kind of
approach of the bot could be very damaging.
I also think that even if we someday succeed in creating a form of synthetic consciousness, it will be inferior to us. The reasons are two folds: we have no Science of Man, we do not understand ourselves. Nor do we have a Science of Consciousness, we cannot even define it!
We seemingly are good at the construction of mechanical and information processing automata. But they are not our peers let alone our superiors. But then, we shall see.
About his other predictions, I wish he had shared with us his chain of (quantitative) reasoning that led him to such numerical probability percentage. He is speculating and his speculations are as good as you or I.
One wonders whether an advanced AI-powered system would not be able, without awareness or emotions as we know them, nevertheless take control and impose its agenda, if only to complete 'the mission' in an uncompromising way. This is what you see in '2001, a Space Odyssey', and other sci-fi films where the robot develops a blueprint of its own, one aim being to continue to exist and another being to deal with problems effectively. Of course, when we say all this, we attribute human characteristics and urges to what are, in essence, IT systems.
In the movie 'Archive': there is an interesting
take on this, but quite far-fetched, clearly. Another interesting movie in this department is 'Ex-Machina'. The films dwell on what it means
to be human. In the case of 'Archive', also, what it means to be alive, and the
ending is quite clever.
Personally, I think it is easy to envision a cybernetic system without emotion or awareness. We already have automated trains, land-vehicles, drone that execute a mission. This concept of mission is already in use in Autonomous Space probes, for example. The mission is a slice of human intentionality, and no one would argue, I think, that the space probe is executing its own agenda.
A time-bomb, another kind of automaton, would explode and it has neither awareness nor knowledge. But let us consider a single-cell organism such as an amoeba.
An amoeba is clearly executing its own agenda even though, we are currently unwilling to attribute to it any intentionality, or awareness. It has a mission to survive (Spinoza would call it Conatus) and maintain itself. It has no awareness (let us say, until proven otherwise) but it follows its mission with awareness or emotion. In my opinion, here, the cell, its mission (to continue to metabolize food and to reproduce) are one and the same.
(These
are philosophical questions if an amoeba has awareness, has
self-awareness, has emotion. They are philosophical questions since we
only are aware of those things in ourselves with confidence. We extend
those things to dogs, let us say, but beyond birds, our confidence starts going
down...)
So, can we build something like an amoeba? I read that there have been synthetic single-cell life forms that have been created by using pieces of extant single cell organisms. But nothing has ever been built starting with raw chemicals. And if extant single cell organisms are used, per my point above, it has the same mission as others. For example, could we create a single cell organism whose mission was just to eat and not to procreate? I do not know but I do not think we know how to do that from ground up, we have no Science of Life.
My ruminations about amoeba were meant to clarify that the Amoeba's agenda is woven into its structure, and we do not know how it came about. We have no knowledge of that, just fancy narrative about "Evolution", with which, just like Jesus, all things are possible.
I think what people seem to be concerned about is the creation of an artificial system with a Self. Again, our first problem is how to define Self. Our second problem is how to relate "Self" to something external to it. (In Newtonian mechanics, Force is an intuitive human-based notion that is related to external events via Newton's Second Law. In Newtonian mechanics, Force drops out of calculation, e.g. in calculating planetary orbits, for it has served its purpose.)
Yes, so, if we know how to create a self, how to endow it with awareness, how to give it the ability to alter itself, and give it either an Agenda or to generate its own Agenda, etc., we could be in trouble because such a system, like H.A.L., could be unstable, mad, deranged....And again, I come back to the same basic question: Where is the Science of Man, in a manner analogous to the Science of Mechanical engineering, that permits us to design land vehicles, or bridges, or aircraft?
We cannot cure mental patients because we have no Science of the Mind even though there are competing un-empricial rationalistic models of the mind proposed by Freud, Jung, Piaget and others. We have no generic Science of the Mind that we could specialize to cats, let us say, or to a Termite Colony. In the absence of such sciences, we have, in A.I., a collection of heuristics, techniques, and specialized applications. When a translator produces text from Persian to English, it does imply that the translator understands those sentences.
When I worked on Solar Ponds, my supervisor told me that sometimes he feels that pond was acting like a living being. I think that was probably because the pond was acting in a non-linear way. And perhaps we have a tendency to equate nonlinear phenomena with Life, which is the epitome of nonlinearity. Perhaps something like this is going on with (generative) A.I. and large language models as well, the output is nonlinear, and we like to endow it with consciousness.
Lastly, I think we have another aspect of human emotion involved here, i.e. Womb Envy by all these purveyors AI: "Look, Ma, I could procreate without a woman!". Since, dealing with women, as explained by Wolfram von Eschenbauch in Parsifal, is not easy.
---------------------------------------------------------------------------------------------------------------------------
Friday, December 27, 2024
Monday, December 23, 2024
Saturday, December 21, 2024
How the Creative Minds Come by their Ideas
From the book:
The
Creative Mind: Myths and Mechanisms
By Margaret A. Boden, Research Professor of Cognitive
Science
Ellipsometry for Autonomous Vehicles
Introduction
Ellipsometry consists of the measurement of the change in polarization state of a beam of light upon reflection from the sample of interest. The exact nature of the polarization change is determined by the sample's properties (thickness and refractive index). The experimental data are usually expressed as two parameters Ψ and Δ. The polarization state of the light incident upon the sample may be decomposed into an s and a p component (the s-component is oscillating parallel to the sample surface, and the p-component is oscillating parallel to the plane of incidence).
The intensity of the s and p component, after reflection, are denoted by Rs and Rp. The fundamental equation of ellipsometry is then written:
Polarimetry and Vehicle Paint
Vehicle Paint consists of multiple layers of thin
films. Please see below.
Schematic of a typical four-layer automotive coating system.
At the first approximation, the quantity ρ in the fundamental equation of ellipsometry above would be a function of refractive index n, extinction coefficient κ and the thickness d of the Clearcoat. Note that n and κ are frequency-dependent parameters. That means that the quantity ρ will have different values at different frequencies for different sensor types.
(For multi-layer
films, a more detailed treatment may be found in: "Simultaneous
measurement of the refractive index and thickness of thin films by S-polarized
reflectances" by Tami Kihara and Kiyoshi Yokomori, Applied Optics Vol. 31,
Issue 22, pp. 4482-4487 (1992) https://doi.org/10.1364/AO.31.004482).
Application to Autonomous Vehicles
Autonomous vehicles are equipped with multiple sensors that
operate in different ranges of the electromagnetic spectrum. These include LiDAR, RADAR, Cameras, and
Infrared sensors. The basic idea is to
characterize the polarization signature of GM vehicles by measuring the
quantity ρ at two locations: at the vehicle during test and development of that
vehicle make and model and inside the AV vehicle during its operations to infer
if an object is a vehicle or not.
The steps of this approach are as follows:
Step 1
- Camera: Measure ρ in the visible light range: 7.5 exp (14) Hz. To 4.3 exp (14) Hz.
- LiDAR: Measure ρ at 905 nanometer and 1550 nanometer
- RADAR: Measure ρ in the range automotive band: of 76 GHz. to 81 GHz.
- Infrared: Measure ρ in the range of 300 GHz. to 430 THz.
Step 2
- For each sensor type in the vehicle, develop processing logic to measure the quantity ρ from scattered electromagnetic signals that are received.
- For each sensor type (LiDAR, RADAR, Camera, IR) cluster the values that have been computed in the previous sub-step. This will give a spatial distribution of the quantity ρ for each sensor type. This spatial distribution is a proxy for the object that is being detected.
- For each sensor type (and the corresponding frequency range), using statistical or other Machine Learning techniques, match the values of ρ against those obtained in Step 1. Use those values, singly or as part of a voting scheme, and in conjunction with other forms of analysis (i.e., ANN) to infer if the signals detected by the sensors are coming from a vehicle or not.
Other Steps
- Work with other OEMs to get their polarization signatures added to the internal models of AV.
- Test and validate there are a need for a calibration step as well as a need to model sensor degradation over time for this sort of applications.
- Use nanoparticles in the paint to enhance the polarization signal and give a specific GM-signature.
Friday, December 20, 2024
Detroit Area IT Contracting Companies
Here are some contract companies in the Metro Detroit:
IT Staffing and Consulting Firms
- TEKsystems
- Description: Provides
IT staffing, talent management, and services.
- Website: TEKsystems
- Contact: Detroit office -
(248) 728-1200
- Kelly Services
- Description: Offers workforce
solutions, including IT staffing and outsourcing.
- Website: Kelly Services
- Contact: Troy office - (248)
362-4444
- Collabera
- Description: Specializes in
IT staffing and professional services.
- Website: Collabera
- Contact: Detroit office -
(877) 264-6424
- Modis
- Description: Provides
IT staffing, consulting, and project services.
- Website: Modis
- Contact: Southfield office -
(248) 351-5450
Consulting and Professional Services Firms
- Capgemini
- Description: Global leader in
consulting, technology services, and digital transformation.
- Website: Capgemini
- Contact: Southfield office -
(248) 936-0000
- Accenture
- Description: Provides strategy,
consulting, digital, technology, and operations services.
- Website: Accenture
- Contact: Detroit office -
(313) 471-3800
- Cognizant
- Description: Offers
IT consulting, digital, technology, and operations services.
- Website: Cognizant
- Contact: Detroit office -
(313) 300-0441
Engineering and Technology Services Firms
- Altair
- Description: Provides software and
cloud solutions in simulation, IoT, and AI.
- Website: Altair
- Contact: Troy office - (248)
614-2400
- Infosys
- Description: Offers business
consulting, IT services, and outsourcing.
- Website: Infosys
- Contact: Detroit office -
(248) 727-4500
- Harman International
- Description: Provides connected
products, automotive, audio, and enterprise solutions.
- Website: Harman
- Contact: Novi office - (248)
474-5200
Saturday, December 14, 2024
News of COVID-19 mRNA Vaccines
https://pubmed.ncbi.nlm.nih.gov/38583833/
Friday, December 13, 2024
Building an AI/ML Risk Analyzer - A Proposal
Summary:
As the application of Artificial
Intelligence (AI) and Machine Learning (ML) algorithms spread in the automotive
domain (as well as other areas of business and industry), it is very important
to be able to assess the risks that inhere in those algorithms in order to
avoid or to minimize those risks. Specially in the automotive domain, it
is critical to mitigate or to eliminate threats to passenger safety, security,
and comfort.
AI/ML algorithms are often presented
in pseudo-code and/or are implemented in a high-level programming language such
as Python, Java, C#, C++. It is desirable to have a software system that
automatically assesses the robustness of an AI/ML algorithm against several
risk scenarios, identify them, categorize them, and supplies remediation
approaches.
Risk Score Calculation
The invention is envisioned as
automated process which analyzes an algorithm for risks inhering in it and
recommends remediation steps. The invention processes different data
sets sequentially for the same trained model. That is, it keeps the
trained algorithm constant and exercises it against different data sets.
The invention performs the following steps (per)
Figure 1
- It will perform a variety of pre-processing steps such
as checking the dimensions of the input vector and the dimension of the
output vector (feature space). In such an example, if dimension_of_input
< dimension_of_output then the model is risky and the system will
so advise the user. Other such pre-processing steps could be added
for different AI/ML algorithms. These pre-processing checks are
configurable by the user of the system.
- It will compute a data difference score by a
suitable method of data subtraction (there could be many such methods –
simple XOR, CRC difference, etc. These methods, for each data set
and algorithms, can be defined via a Data Difference Editor feature of the
invention.)
- The run-time – Process Orchestrator – will execute the
algorithm and compute its Risk score.
- It will then subtract the new Risk Score from the
Trained-Model’s Risk Score.
- Did it increase – by what percentage?
- Conceivably, given a lot of data sets that are
different, one can even generate good statistics for the Risk Score
changes.
- So, we can compute an ensemble average of the change in
Risk Score and then proceed to the TRIZ steps.
The Orchestrator is programmed to
process a list of data sets until the list is exhausted. As a Workflow Orchestrator, we will be using a tool such
as ML Flow since it provides a pipeline for AI/ML algorithms. It executes
the algorithms and gathers performance metrics. Suitable software
components would then analyze those performance parameters for Risks. The
invention is not dependent on ML Flow, any other Workflow Orchestrator
would work as well.
Figure 2
A trained AI model (to be analyzed)
is fed with an specific dataset on which the model has been trained and
validated. The following is a partial list of performance parameters that could
be evaluated in order to combine them, via the Risk Score Editor, into a
Risk Score.
- Accuracy
- True Positive Rate (TPR)
- False Positive Rate (FPR)
- Recall
- Precision
- F1-Measure
- Area under the Receiver Operating Characteristic (ROC)
curve
- Area under the Precision-Recall (PR) curve
- Logarithmic loss
- C-Measure
- R-Measure
An example of performance parameters
for Classical Binary Classification is displayed below - analogues of such
parameters can be defined for other AI/ML algorithm types.
Figure 3
Those are captured in
the Algorithm Types & Performance Parameters data store.
This invention is intended for
mitigating the risks of the trained model and not the data. The main
objective of this tool is to advise the modeler to mitigate model-specific
issues before going to Deployment phase of the model. Its output could be
used to indicate the robustness of a trained model with respect to data
differences.
Generating Risk Remediation Hints
Risk is estimated based on a
combination of performance parameters. The System will go through
performance parameters - based on the "strength" of that parameter in
the calculation of the combined Risk Score and supplies hints to the algorithm
designer.
The way that is done is that the
Risk Score is disambiguated by looking at its parts - per its definition via the Risk Score Editor
(please see below).
The system utilizes the validated TRIZ approach.
Figure 4
TRIZ principles are
not changed but their analogue realizations in AI/ML domain are used.
There are 39 technical parameters
(or features) in TRIZ. To use the TRIZ contradiction matrix, the performance
parameters of AI/ML algorithms are mapped to the closest TRIZ equivalent
feature. They are then placed at the same position of their equivalents
in the TRIZ contradiction matrix, and then the system uses the
suggested TRIZ inventive principles to resolve the tradeoff between pairs
of AI/ML features.
AI/ML Analogue of TRIZ General
Attributes
Below is table of TRIZ general
system attributes / parameters that with their analogues of Attributes for
AI/ML.
Attribute |
Interpretation |
Possible Contrivances |
AIML Example(s) |
Speed |
|
|
|
Strength |
|
|
|
Loss of Information |
|
|
|
Quantity of Substance / Matter |
|
|
|
Reliability |
|
|
|
Accuracy |
|
|
|
Adaptability / Versatility |
|
|
|
Productivity |
|
|
|
Once the TRIZ principle is
identified, a hint or a number of hints could be generated from the TRIZ-Hints
data.
A Partial Realization of
TRIZ-supplied Hints Table is presented below:
TRIZ Principle |
AI/ML Hint |
|
1 |
Segmentation /Division |
Refactor the Algorithm into many independent algorithms. |
3 |
Local Quality |
Non-uniform data sampling method |
5 |
Consolidation/Combination/Merging |
Data Dimension Reduction (PCA / Autoencoding) |
6 |
Universality /Multi-function |
Parallelize the computation |
17 |
Transition into New Dimension/Another
Dimension |
Change the number of layers (ANN) One-hot-encoding PCA analysis |
The system will use a pre-populated
Risks Map to identify the corresponding record in a TRIZ-like Contradiction
Matrix for => Hints
Examples of TRIZ-like contradictions
are
- performance parameters vs speed of execution
- precision vs numerical stability
The inventions contain the following
"editors":
Data Difference Editor
This enables a user of the system to
define how to compute the difference between the training set of data for a
model and those that will be used during the process of Risk Assessment by the
Process Orchestrator. There are many well-known such methods in prior art such
as make use of cyclic redundancy checks (CRC) for each data set, XOR Operation,
Image Subtraction, comparing topological invariants of each data set and very
many others.
It should be noted that the data
types one deals with is, in practice, finite. We have:
- Relation Data (Structured Data - use binary
subtractions? - attribute by attribute subtraction).
- Textual Data
- Different types of images (enabling the usage of Image
Analysis techniques)
- Camera
- Lidar
- Radar
- Different Types of Sensors (time series - using
mathematical statistical techniques)
- Temperature Sensor (infrared)
- Proximity Sensors
- Infrared Sensor (IR Sensor)
- Ultrasonic Sensor
- Light Sensor
- Smoke and Gas Sensors
- Alcohol Sensor
- Touch Sensor
- Color Sensor
- Humidity Sensor
- Tilt Sensor
Furthermore, the number of sensor
types is limited by the Laws of Physics and Chemistry and therefore is finite
as well.
Given that the data types are
finite, we can use existing measures (Known in the Arts) to characterize the
difference between a training dataset and other datasets during the execution
of an AI/ML algorithm by the Process Orchestrator.
For example, for time-series sensor
data, we have metrics such as the mean, the standard deviation of the mean, the
median, min/max, and the power spectrum to utilize in order to compute the
difference between the reference dataset used in training and those fed to the
algorithm via the process orchestrator.
For image type sensor data (Camera,
Lidar, Radar), which occupy different parts of the electromagnetic spectrum,
many available image analysis techniques could be used to compute the dataset
difference.
In principle, a combination of
metrics could be applied to measure the differences between the training
dataset and those used during the execution of the model by the Process
Orchestrator.
A combined difference score is also
computed if the user so decides via this editor.
Risk Score Editor
This enables a user of the system to
define the Risk Score for an algorithm based on its performance parameters. The
user has a lot of flexibility on the ways to combine the performance parameter
of a given model to create a Risk Score for that algorithm; i.e. giving each
parameter a different weight and compute the arithmetic average of those
parameters. The system will output Risk Score and a Probability of that risk
(its confidence level). The Risk Score will be a Real number between 0 to 1.0
and the thresholding (low, medium, high) would be based on the criticality of
the application (safety, money, health) Threshold is the user selection.
The Risk probability would be computed based on the ratio of occurrences of a
Risk situation (defined as when small changes in data lead to large changes in
the Risk Score) to the total number of Risk Scores that are calculated.
Algorithms List Editor
The system is fitted with a
pre-populated data store of AI/ML Algorithms and their associated performance
parameters. This list can be updated via this Algorithms list Editor in
order to specify new algorithms and their corresponding performance parameters.
The important point is that this is a finite list of AI/ML Algorithm
types which is extensible and maintainable.
Active Reinforcement
Learning |
Local Search in Continuous
Spaces |
Agents Based on Propositional Logic |
Machine
Translation |
Alpha-Beta
Pruning |
Multiagent
Planning |
Artificial Neural
Networks |
Nonparametric
Models |
Augmented Grammars and Semantic
Interpretation |
Object Recognition by
Appearance |
Backtracking Search for Constraint Satisfaction
Problems |
Object Recognition from Structural
Information |
Bayesian
Networks |
Ontological
Engineering |
Complex Decisions - Policy
Iteration |
Optimal Decisions in
Games |
Complex Decisions - Value
Iteration |
Partially Observable
Games |
Constraint Propagation: Inference in Constraint
Satisfaction
Problems |
Passive Reinforcement
Learning |
Decision
Networks |
Planning and Acting in Nondeterministic
Domains |
Dynamic Bayesian
Networks |
Planning Graphs as State-Space
Search |
Ensemble
Learning |
Problem-Solving
Agents |
Explanation-Based
Learning |
Propositional Theorem
Proving |
First-Order Logic with Backward
Chaining |
Reconstructing the D
World |
First-Order Logic with Forward
Chaining |
Regression and Classification with Linear
Models |
Heuristic Functions |
Relational and First-Order Probability
Models |
Hidden Markov
Models |
Robotic Moving |
Imperfect Real-Time
Decisions |
Robotic
Perception |
Inductive Logic
Programming |
Robotic Planning to
Move |
Information
Extraction |
Robotic Planning Uncertain
Movements |
Information
Retrieval |
Searching with Nondeterministic
Actions |
Informed (Heuristic) Search
Strategies |
Searching with Partial
Observations |
Kalman Filters |
Sequential Decision
Problems |
Knowledge Engineering in First-Order
Logic |
Speech
Recognition |
Learning Decision
Trees |
Statistical
Learning |
Learning Using Relevance
Information |
Stochastic
Games |
Learning with Complete
Data |
Supervised Learning |
Learning with Hidden Variables: The EM
Algorithm |
Support Vector
Machines |
Local Search Algorithms and Optimization
Problems |
Syntactic Analysis (Parsing) |
Local Search for Constraint Satisfaction
Problems |
Text
Classification |
Uninformed Search
Strategies |
TRIZ Attributes Editor
Mapping qualitative attributes to
quantitative metrics is using an application of the House of
Quality concept to this invention.
It is intended as a tool to help us
map TRIZ attributes into quantitative measure of the type of AI/ML algorithms.
The user will select the TRIZ
attributes that are relevant to an specific AI/ML algorithm.
The user thresholds the performance
parameters; i.e. if threshold is not met, find the relevant attribute, and go
back to the TRIZ contradiction matrix and look for hints.
Please note that the Risk Score is a
combination of these performance parameters below:
Figure 5
Key Idea
The key insight of the invention is
to measure - in a suitable and configurable manner, as specified by the AI/ML
Algorithm designer - the Risk Score of a trained AI/ML model by subjecting it
to a plurality of of real or simulated or
spiked data sets via an automated process.
The basic idea is that
small differences between the training (golden) data set and a plurality of
similar data sets must be accompanied by small difference of the model's Risk
Score.
The word small is something to be
defined per algorithm. Small & Large changes do not mean changes in the
pixels alone, but other factors - say color, or other geometrical properties,
e.g. number of holes. The test data sets could be generated by adding noise to
the data, or other objects, rotating the data in the space of similar such
data, and so on.
Another key novelty of this system
is its use of TRIZ principles as applied to AI/ML Algorithms.
A third novelty of this invention is
that it can also assess the stability of an AI/ML Algorithm by comparing
changes in the input data (from the training data) with corresponding changes
in the performance of that algorithm.
Useful Links
- .Net Code Samples
- AJAX for MS Developers
- C# Tutorials
- Channel9
- Code Search Engine
- Douglas Crockford's JavaScript Site
- DZONE
- Google Code
- IBM Developer Works
- IBM Public Skunkworks
- Is This Thing On?
- Java tutorials, hints, tips
- Jon Udell Weblog
- Knowing .Net
- Massive List of Information for Programmers
- MIT Courses
- MSDN
- Simple-Talk
- SUN Java
- That Indigo Girl
- UC Berkeley Lectures
- Yahoo UI Library
Topics
- 3-D Printing (14)
- AI (318)
- Art (109)
- Article (142)
- book (14)
- books (84)
- Business Intelligence (18)
- Careers (91)
- Cloud Computing (20)
- Cognition (13)
- Complexity (8)
- Computer Science (22)
- COVID-19 (1)
- Cyber-security (83)
- Data Analysis (41)
- Data Management (21)
- Data Visualization (31)
- Design Thinking (1)
- Embedded Tools (34)
- Gadgets (76)
- Games (33)
- Google (8)
- Hardware (44)
- High Performance Computing (33)
- History of Mathematics (1)
- Humor (77)
- Inetrview (7)
- Intelligent Transportation (17)
- IoT (15)
- IT as Metaphor (2)
- Magazine Subscription (8)
- Mathematics Tools (4)
- Microsoft Platforms (23)
- Microsoft Tools (65)
- Mobile Computing (3)
- Motto (3)
- Network Tools (12)
- News (161)
- Offshoring (6)
- Open-Source Sofware (9)
- Outsourcing (1)
- Philosophy (7)
- picture (1)
- Pictures (149)
- PLM (5)
- Programming Languages (75)
- Quantum Computing (5)
- Reports (53)
- RFID (3)
- Robo (2)
- Robots (111)
- Science (65)
- Scientific Computing (18)
- Search Tools (7)
- Semantic Networks (11)
- Simulations (34)
- Social Computing (26)
- Software Architecture (27)
- Software Development (161)
- Software Testing (5)
- Software Tools (271)
- Some Thoughts (64)
- Speech (6)
- Standards - Telematics (9)
- Transportation (14)
- Video (11)
- Visualization (10)
- Web Site (233)
- Web Site for Science (51)
About Me
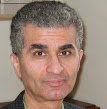
- Babak Makkinejad
- I had been a senior software developer working for HP and GM. I am interested in intelligent and scientific computing. I am passionate about computers as enablers for human imagination. The contents of this site are not in any way, shape, or form endorsed, approved, or otherwise authorized by HP, its subsidiaries, or its officers and shareholders.
Blog Archive
- July (4)
- June (15)
- May (15)
- April (9)
- March (17)
- February (22)
- January (45)
- December (19)
- November (11)
- October (10)
- September (7)
- August (11)
- July (6)
- June (11)
- May (12)
- April (7)
- March (5)
- February (1)
- January (3)
- December (1)
- October (2)
- September (4)
- August (1)
- July (3)
- June (2)
- April (2)
- March (2)
- February (2)
- January (10)
- December (1)
- October (1)
- September (1)
- August (4)
- June (1)
- April (6)
- March (2)
- February (4)
- January (3)
- December (1)
- October (1)
- June (3)
- April (1)
- March (1)
- February (1)
- January (6)
- December (8)
- November (3)
- October (5)
- September (2)
- August (3)
- July (6)
- June (2)
- May (7)
- April (19)
- March (22)
- February (6)
- January (5)
- December (4)
- November (4)
- October (9)
- September (3)
- August (7)
- July (3)
- June (2)
- May (6)
- April (4)
- March (8)
- February (5)
- January (18)
- December (6)
- November (10)
- October (6)
- September (7)
- August (2)
- July (4)
- June (5)
- May (8)
- April (5)
- March (9)
- February (3)
- January (7)
- December (2)
- November (1)
- October (3)
- September (5)
- August (10)
- July (8)
- May (5)
- April (8)
- March (9)
- February (6)
- January (11)
- November (6)
- October (9)
- September (5)
- August (13)
- July (9)
- June (9)
- May (8)
- April (4)
- March (2)
- February (8)
- January (9)
- December (3)
- November (7)
- October (9)
- September (7)
- August (4)
- July (2)
- June (4)
- May (7)
- March (4)
- February (2)
- January (1)
- December (2)
- November (1)
- October (6)
- September (1)
- August (1)
- July (4)
- June (1)
- April (1)
- March (1)
- February (1)
- January (2)
- December (5)
- October (4)
- August (2)
- July (3)
- June (8)
- May (7)
- April (5)
- March (9)
- February (3)
- January (7)
- December (4)
- October (7)
- September (5)
- August (5)
- July (8)
- June (6)
- May (9)
- April (5)
- March (4)
- February (5)
- January (6)
- December (12)
- November (7)
- October (5)
- September (4)
- August (19)
- July (12)
- June (4)
- May (8)
- April (5)
- March (15)
- February (5)
- January (9)
- December (14)
- November (6)
- October (12)
- September (2)
- August (10)
- July (8)
- June (8)
- May (11)
- April (10)
- March (10)
- February (9)
- January (20)
- December (16)
- November (9)
- October (25)
- September (24)
- August (12)
- July (18)
- June (20)
- May (13)
- April (29)
- March (26)
- February (14)
- January (17)
- December (17)
- November (9)
- October (32)
- September (27)
- August (27)
- July (11)
- June (22)
- May (25)
- April (33)
- March (33)
- February (28)
- January (38)
- December (12)
- November (39)
- October (28)
- September (29)
- August (29)
- July (18)
- June (27)
- May (17)
- April (23)
- March (40)
- February (31)
- January (6)